- April 12, 2023
- Category: C&Q
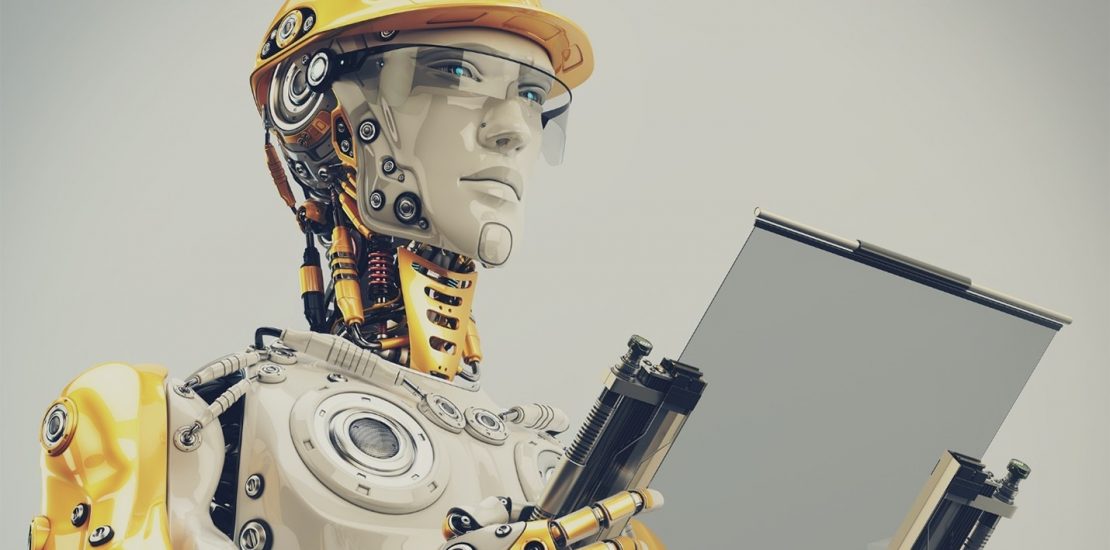
By Bruce Klopfenstein & Viraj Karekar, MMR Consulting
There has been a lot of buzz lately with the rapid emergence of Artificial Intelligence, ChatGPT being the most often referenced, but a quick Google search yields literally hundreds of AI applications being used to aid businesses.
Digital Validation (DV), aka “paperless validation”, has been here for a while. DV of course does more than just digitize paper, it creates validation data that can be used to analyze and develop actionable reporting. Life Science companies are swiftly adopting this more efficient, time-cost-saving approach to commissioning and qualifying their equipment and software.
“Revolutionize” is an over-used term, but the combination of these two quantum leaps in technology applied to the C&Q process will truly deliver on that promise.
There are common phases we go through for a C&Q project, whether we have paper and pencil or the latest DV system. They are:
- The Discovery Phase – Here we examine the client’s Quality System and C&Q procedures to see what gaps, if any, exist between their QMS and the current regulations.
- The Planning Phase – From the procedures, we know what deliverables are required to complete the C&Q effort. With this knowledge, we can build the Deliverables List. We also will review the URS’s and develop a schedule with priorities.
- The Document Development Phase – This is where we create the documents on the Deliverables List and get ready for testing execution once we take ownership of the constructed systems.
- The Execution Phase – Now we’re “in the battle” of reviewing construction, starting up systems, and executing tests. We better have our processes and forms in place and ready as well.
- The Turnover Phase – Finally we will turn over the systems to the site, along with the required documentation. DV & AI will play a big role here as well.
The Discovery Phase – AI reviews client C&Q procedures and looks for gaps in current regulations and guidance.
In the highly regulated world of the Life Sciences industry, ensuring that Commissioning & Qualification procedures are in compliance with current rules and guidance is crucial. That’s one area where AI can help. By automating the review process, AI can help Life Science companies identify gaps in their Commissioning & Qualification procedures and ensure that they meet regulatory standards.
Commissioning & Qualification is a critical step in the development and manufacturing of pharmaceuticals, biologics, medical devices, and other Life Science products. It involves verifying and documenting that equipment, facilities, and systems are installed, operational, and performing as intended. Due to the impact of regulations, any gaps or inconsistencies in Commissioning & Qualification procedures can result in costly delays and potential regulatory penalties.
AI can provide a significant advantage in this area. By leveraging machine learning algorithms and natural language processing, AI can quickly analyze the client’s Commissioning & Qualification procedures and identify any areas that may be non-compliant or out of date with current laws and guidance. This can help Life Science companies to proactively address any issues before they become a problem, saving time and money in the long run.
AI uses this gap analysis to make recommendations for SOP modifications and updates. The recommendations can take the form of tracked changes in the procedures which can be reviewed and accepted, deleted, or modified.
Transitioning to the updated approach
AI can also assist an organization transitioning to the new commissioning and qualification approach by providing training modules and new procedures.
- Analyzing and understanding the new approach: AI can analyze the new commissioning and qualification approach to understand its key principles, requirements, and objectives.
- Developing training modules: AI can help develop training modules to help personnel understand the new approach, including key concepts, best practices, and procedures. These training modules can be customized for different roles and responsibilities and can be delivered through various channels, such as e-learning, virtual reality, and interactive simulations.
- Developing new procedures: If required, AI can help develop new procedures and guidelines to align with the new commissioning and qualification approach.
- Monitoring and optimizing the transition: AI can monitor the progress of the transition and identify areas that require further attention or improvement. It can also analyze data and feedback to identify areas where the transition process can be optimized or streamlined.
The Planning Phase – Determining the Deliverables List
Artificial intelligence (AI) has the potential to transform the way we manage projects by automating tedious and time-consuming tasks. One such task is the review of a large list of systems and determining the required list of document deliverables based on the SOPs to be used for the project. Here’s how AI will achieve this:
First, the AI system will need to be trained on the specific SOPs that govern the project in question. This will involve inputting a large amount of data, including previous project documents, SOP manuals, and other relevant materials. The system will then use machine learning algorithms to identify patterns and relationships between different types of documents and the SOPs that govern them.
Once the AI system has been trained, it will be able to automatically analyze a large list of systems and determine which documents are required for each one. For example, if a particular system requires a risk assessment, the AI system will be able to identify this based on the relevant SOPs and highlight the need for a risk assessment document.
This list can then be loaded into the Digital Validation (DV) system creating the CQV “to-do” aka “deliverables list” for the project. The DV system will also load the metadata associated with each document such as the system ID, area, criticality, design & equipment data, vendor, etc. If the AI system has knowledge of the client’s organizational data and personnel, it could also provide the DV system with the first cut at the reviewers and approvers for each document.
Overall, the use of AI in reviewing a large list of systems and determining the required list of document deliverables based on SOPs has the potential to greatly improve project management efficiency and reduce the risk of errors or omissions in project documentation.
Determining Document Priority Based on Analysis of the System Dependency Matrix
Often, the C&Q Team is brought into the project later than it should have been. This results in the need to start producing protocols in the order of priority in terms of “which ones do we need first?”. This is best solved using the System Dependency Matrix. It’s an analysis of which manufacturing processes are selected to be started first (chosen by the owner) and working backward to understand the supporting systems.
The System Dependency Matrix is based on which utilities are needed by which processes, including the utilities needed by other utilities, e.g. plant steam is needed to produce heating hot water, but plant steam first needs the condensate system in place; the condensate system needs process drains, etc.
This is a lot of analysis, especially on large, complex greenfield projects. Humans are totally capable of performing this, we do it now, but AI is much more efficient at digesting the P&IDs and determining the priorities. This will allow more what-if scenarios and faster responses to project upsets and changes.
Determining Document Priority based on Analysis of the Current Construction Schedule (Weekly)
The next input into the document priority decision is when the system is mechanically complete. Even if a system shows up later in the Dependency Matrix, if it’s ready to be inspected (starting IV/IQ) and tested (OV/OQ) then the protocols better be ready as well. Again, this can and is done by humans now, but on construction schedules with 10K, 20K – 50K task lines this is a huge effort that must be repeated with every new schedule issue. AI can take data input from the P6 or MS Project schedule, along with document status data from the DV system to determine where the effort needs to be placed by the C&Q Team in the next few weeks. This will avoid having a system mechanically ready for C&Q but lacking the protocols.
Review & Analysis of the URSs and Building the RTM
Writing a good URS is still more art than science at most organizations. The new ISPE C&Q Baseline Guide gives us excellent guidance and examples for this that if applied can help us establish a basis for verifying that the delivered system truly meets the intended use criteria. Load this guidance, along with S.M.A.R.T. testing criteria, templates, and design data into the AI system which will generate a 1st pass URS development that can be loaded into the DV. This 1st pass URS will be sent to the assigned C&Q Team author for review. After updating, the DV will send it to the assigned reviewers earlier identified by AI. Note that the “1st Pass” draft will assign criticality based on the client’s criteria and will also include the logic tying it back to patient safety and quality. This will avoid biased or subjective assignment of criticality, although it can be overridden later in the process by human intervention if desired.
Once the URSs are approved the RTM will be generated by the AI system, using the approved template. DV’s can do this now as it’s mostly mechanical however the next step with the RTM is truly leveraging AI’s potential.
Determining the Leveraging Strategy by Analyzing FAT & SAT Protocols and VDRs Against the RTM
“Leveraging” has been retired from the ISPE C&Q vernacular, as the verification of a system is no longer a series of events (FAT, SAT, IOQ, etc.) but rather one big activity that occurs once the system is defined until it is accepted as “fit for intended use”. However, in what used to be called leveraging, we need to understand where we will find the documentation and testing evidence to prove this final objective. This is a large effort involving the review of different documents and testing protocols, which is where AI shines. AI can not only digest volumes of text in seconds, but it can also determine which evidence to match against the requirements and provide the logic used to justify its decision.
Moving Data from Design through Turnover
Often now we work in silos with each phase developing a great deal of data but without a clear plan for re-using this hard-earned and valuable information. Like C&Q, DV should begin in the Design Phase – collecting design data for use in protocol development. It also provides a basis for setting up the VDRs, aka Vendor Data/Document Requirements, and a recent trend where this is also used to setup CDRs, or Construction Data/Document Requirements. VDRs and CDRs are P.O. based rather than system based but the data is repackaged and becomes part of the system turnover. The review process is often the bottleneck for this process, especially on large projects where the information being received from all parties becomes overwhelming for the engineering team to manage. DV and AI together can manage the fire-hydrant flow of information, verifying that the data/docs received meet the specifications for the P.O., local building codes, and URS for the system.
Determining the CTOP TOC’s
A big advantage for C&Q is to know ahead of time not only the types of documents expected in the system Construction TurnOver Package, or CTOP, but also to know the quantities of each. This can be deduced by reviewing all the P&IDs, noting the system boundary marker locations, taking an inventory of the different components, and then looking up the testing/verification documents required for each of the components. This can take weeks to lay out on a big project. An AI system properly set up and prompted can do this in minutes. And it can prepare the CTOP structure – the Tables of Contents and sections for each system’s CTOP. It could even generate the pre-executed forms to be used by construction in the field and place them in the proper sections. Using the DV system to collect documents executed in the field, the AI system could review these as they are submitted to see if they are filled in correctly and are acceptable per pre-determined criteria.
The Document Development Phase – Drafting Documents
DV systems now help us in developing consistent and comprehensive documentation, but we still must do most of the heavy lifting in terms of content creation. Fortunately, the emergence of artificial intelligence offers a solution that will fill this gap and load DV systems with content-rich documents based on previous steps discussed earlier.
The DV system will begin the document workflow with the C&Q author, who will review the AI-generated draft. This new approach will require most of the C&Q engineer’s time to be spent reviewing these AI-generated documents, prompting the question: how good will they be? However, it’s important to remember that AI has an incredible ability to learn, and learn quickly at that.
If good representations of final documents are loaded into AI, it will learn from them and produce similar efforts. If Best-Practice examples and industry guidance documents are loaded into AI it will study and learn from that as well. It’s what the “P” in GPT stands for – GPT – Generative Pre-Trained Transformer. It also takes feedback (without getting its feelings hurt) and learns from it to provide better responses, and ultimately better documents.
Reviewing and Approving Documents
The DV system will manage the document workflow, versioning, and comment organization but AI can analyze the workflow data to look for trends and patterns to see where the problems are. For example, does a certain author, reviewer or type of system having longer than average cycle times? Are there inconsistencies in how documents are being reviewed or approved? How might additional training or resources affect this? Perhaps with this analysis and knowing the schedule trends (continued slip in construction MC’s for example) re-assignment of documents or reviewers will result in project savings. AI could also generate schedules for reviewers and approvers based on its knowledge of the construction schedule and the DV’s document status. It could also analyze their workload to see if those resources are correctly staffed to meet the timeline.
The Execution Phase – Protocol Field Completion
DV is built to manage this effort – field execution, workflow, completion status, evidence collection, etc., again where AI comes in to complement this process is by analyzing the data generated by the DV system complemented with data from the other project systems. AI will have the current project knowledge due to its earlier involvement; it can also have been fed previous similar projects to see how this project is performing in comparison. It can look at patterns and trends in execution times to predict issues and recommend possible solutions. It would basically be doing what an experienced C&Q Lead does intuitively, but with hard data and unlimited time in terms of computer cycles. Obviously, this would need to be set up – the right data loaded, and the right prompts fed into the AI system. This will take time initially as we begin experimenting with AI, but as the technology continues to develop, we can expect to see even more advanced systems that are capable of handling increasingly complex tasks like this.
The Turnover Phase – Review & Analysis of the Completed Protocols and Writing Reports
Once the DV system indicates that the protocol is complete, AI can review the content. Depending on how the test scripts are written (again a DV function) it can add conclusions or summaries, or just verify values. It can assist with discrepancy writeups and recommendations based on previous writeups or via analysis of company procedures and practices. It can also write acceptance reports for a completed system. As in protocol development, the DV system would manage the document workflow, starting with the C&Q author/owner of the protocol who will review any AI-generated content before being sent to the client.
Conclusion
As of late, Artificial Intelligence (AI) has been making significant progress. However, it still has a way to go before it can be fully trusted to provide final documentation for Life Science and other regulated industries. Although AI can produce drafts, they must be reviewed by qualified individuals before they can be utilized. Nonetheless, using AI to generate drafts and outlines can significantly reduce overall project effort. The same applies to AI data analysis, which, like any software, must be validated before it can be commercially utilized without constant review. Nevertheless, the capabilities of AI go beyond using spreadsheets or code; it can create the necessary spreadsheet or code and even produce text that explains the results obtained. While proper application and management are necessary, this technology is currently available and has the potential to revolutionize the way we work.
As we move towards the digital age, the future of validation is set to undergo a radical transformation. The days of cumbersome paperwork and manual processes are soon to be a thing of the past. Instead, we can expect to see a fully digital validation process that relies on cutting-edge technologies such as digital design documents, digital TOPs, and digital CQV software.
But that’s not all. The future of validation will also incorporate the power of AI, allowing for increased efficiency, accuracy, and speed. And with all parties collectively working in the digital software, including equipment vendors, AE firms, automation firms, owners, and owners’ CQV agent/team, we can expect a truly collaborative and streamlined approach to validation.
The benefits of this digital revolution are clear. Not only will it reduce the risk of errors and delays, but it will also save time and money. As we look towards the future of validation, it’s also clear that the digital transformation is well underway, and those who embrace it will undoubtedly reap the rewards.
As a final note, it is often stated that AI will not replace human jobs, but rather, individuals utilizing AI will replace human workers. Similarly, AI will not replace companies; instead, companies that adopt AI will outperform and surpass those that do not. The competitive advantage that AI can provide is too significant to be ignored, and organizations that do not utilize it risk being replaced by those that do.
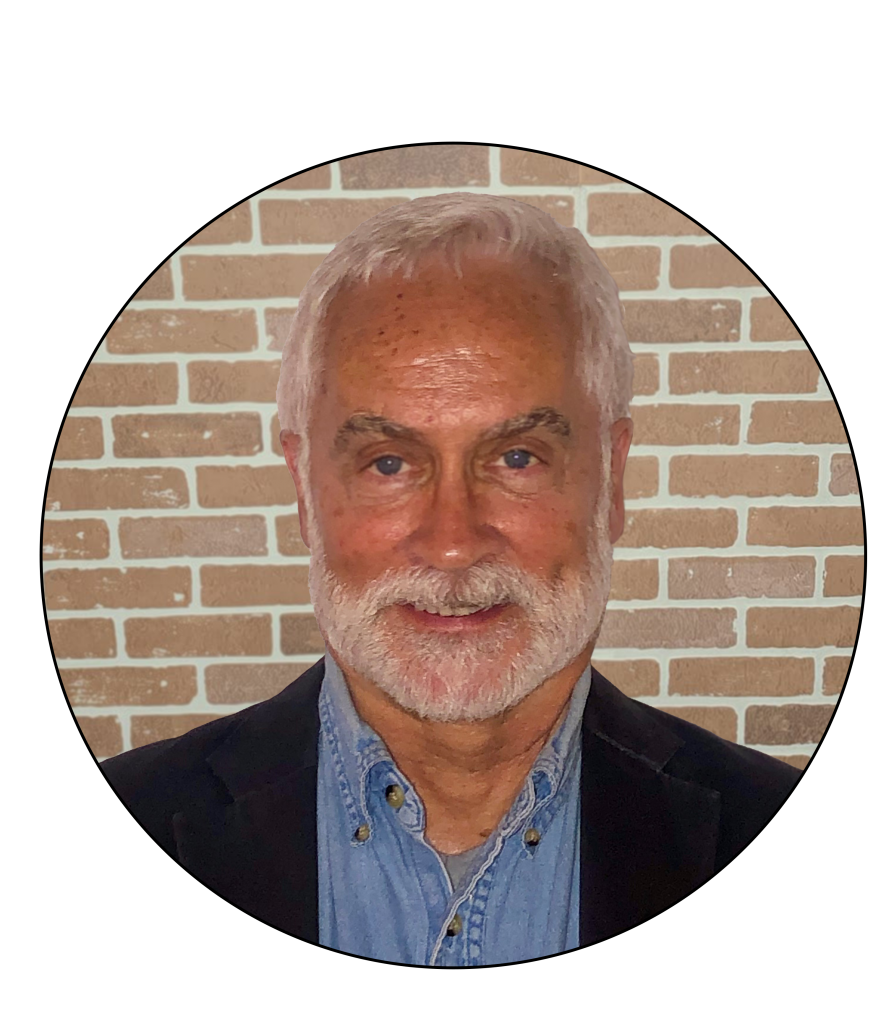
Written By
Bruce Klopfenstein
CQV SME
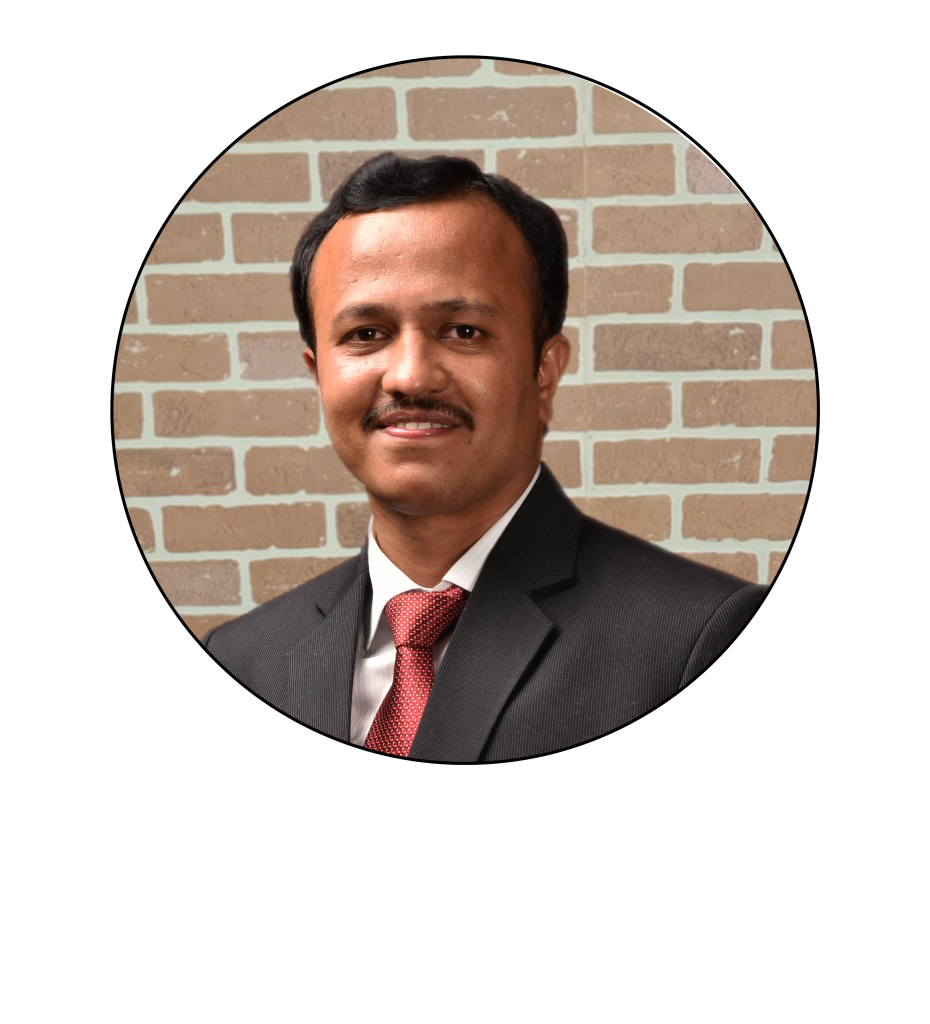
Written By
Viraj Karekar
C&Q Manager